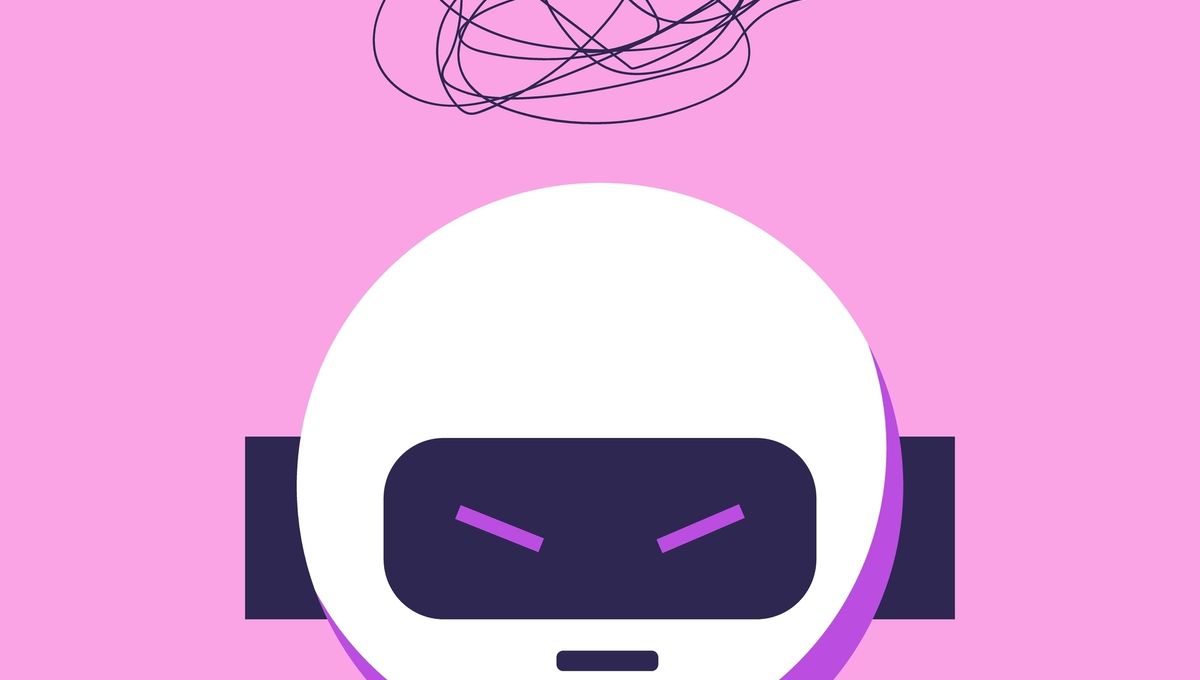
While AI may be more commonly used for stealing art and hallucinating bullshit – that’s a technical term, by the way – the last couple of years have also seen what seem to be some genuinely extraordinary feats from the nascent technology. And that’s particularly true in the field of math: where computers were once confined to the category of blunt force instruments, today they can apparently not just solve complex problems, but can come up with novel proof strategies all of their own.
But just how smart are they, really? In a new paper, expert mathematicians set forth a new challenge for today’s top level AI programs. The result? Abject failure.
“Recent AI systems have demonstrated remarkable proficiency in tackling challenging mathematical tasks, from achieving olympiad-level performance in geometry to improving upon existing research results in combinatorics,” begins the paper, currently published on the ArXiv preprint server. “However, existing benchmarks face some limitations.”
For example, the authors write, while it’s certainly impressive that AI systems can tackle challenges like the GSM8K problem set or the International Mathematical Olympiad, neither of those are exactly cutting-edge math – they’re more like “advanced high school” level than “limit of human invention”.
On top of that – and also reminiscent of high school math – we’re running out of things to ask our various AI programs. “A significant challenge in evaluating large language models (LLMs) is data contamination,” the authors explain – in other words, “the inadvertent inclusion of benchmark problems in training data.”
Like a student acing a test they already saw the answer key to, “this issue leads to artificially inflated performance metrics that mask models’ true reasoning capabilities,” they write.
The solution: FrontierMath – described by the team as “a benchmark of original, exceptionally challenging mathematical problems created in collaboration with over 60 mathematicians from leading institutions.” It’s no empty boast: there are multiple Fields Medal winners involved in the project, including one who contributed problems to the dataset; other tests came from mathematicians of graduate level and up, from universities across the world.
Problems submitted had to meet four criteria: they had to be original – to “[ensure] that solving them requires genuine mathematical insight rather than pattern matching against known problems,” the paper explains; they had to be guessproof; they had to be “computationally tractable” – that is, they had to be relatively straightforward if you know what you’re doing; and they had to be quickly and automatically verifiable. Once all these boxes were checked, the questions were even peer-reviewed, rated for difficulty, and handled securely to prevent dataset contamination.
It was, in other words, no small feat. But could today’s AI programs beat it?
Well… no. “Current state-of-the-art AI models solve[d] under 2 percent of problems,” the authors write, “revealing a vast gap between AI capabilities and the prowess of the mathematical community.”
Now, AI shouldn’t take this too hard – the problems were very difficult. “[They] are extremely challenging,” Fields Medal winner Terence Tao said, requiring extensive training data that is, in practice, “almost nonexistent.”
But it does mean that, for now at least, the FrontierMath dataset is kind of hoisted by its own petard. “Current AI models cannot solve even a small fraction of the problems in our benchmark,” the authors write. “While this demonstrates the high difficulty level of our problems, it temporarily limits FrontierMath’s usefulness in evaluating relative performance of models.”
“However, we expect this limitation to resolve as AI systems improve,” they add.
The paper – which includes sample problems and solutions from the dataset – is published on the ArXiv.
Source Link: Today's Top AI Went Up Against Expert Mathematicians. It Lost Badly.